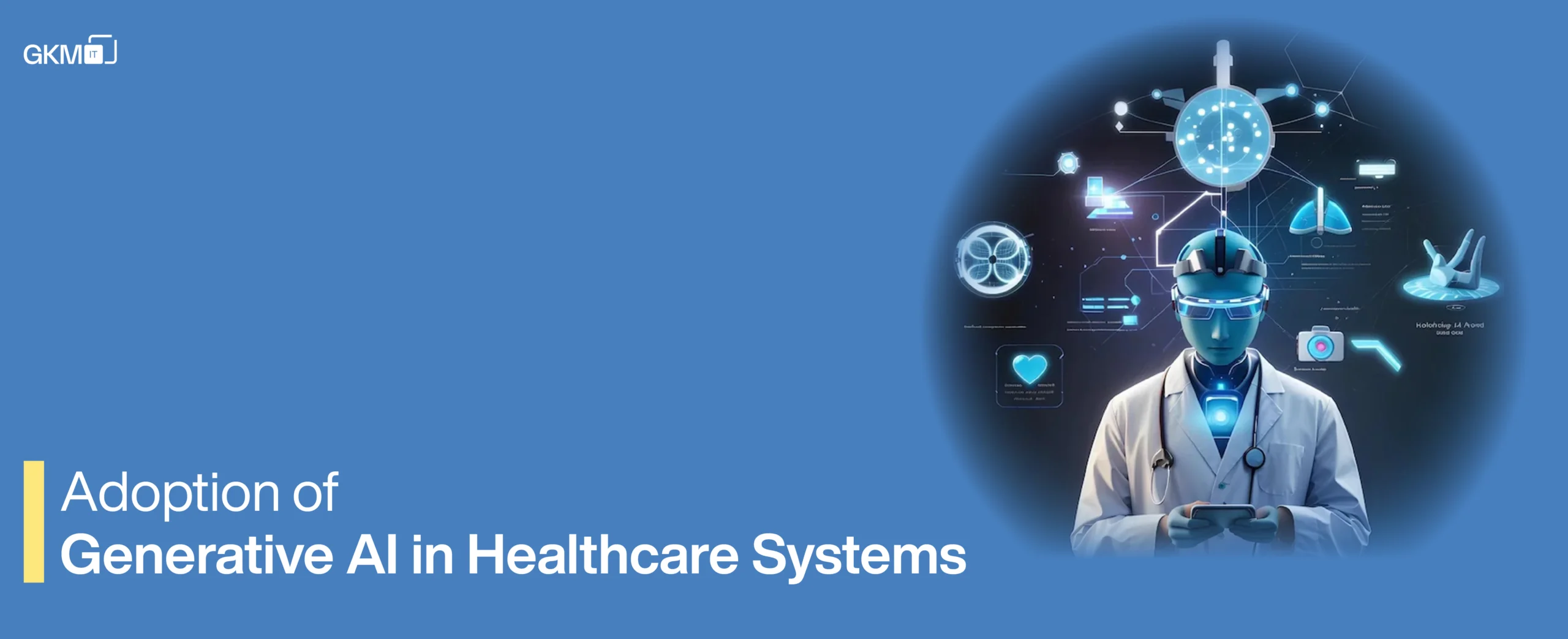
Adoption of Generative AI in Healthcare Systems
The healthcare industry is going through a revolutionary change, primarily inspired by Artificial Intelligence (AI). Of all these innovations, Generative AI or GenAI in the healthcare industry can be considered as a revolution. In this way, Generative AI that allows machines to generate new valuable data has incredible potential in healthcare applications ranging from diagnostics to drug development and individualized treatment. Such an adoption is especially apparent in use cases like medical imaging, clinical decision support systems, and synthetic healthcare data creation.
What is Generative AI in Healthcare?
Generative AI is a machine learning model that can generate new data throughout the existing data set. In contrast with classical AI, which was aimed at data analysis and processing, it can generate new solutions, content, or designs. In healthcare, this can be anything from creating medical images to creating treatment plans or finding new drugs.
Another emerging interest in the healthcare field is to use AI in the healthcare system, which provides a solution that is effective, precise, and can be enlarged to treat patients’ data, research, and health services. Another area in which AI is revolutionizing the healthcare sector is the application of AIOps. AIOps in healthcare is integrating artificial intelligence with operations to make healthcare clinical and operational processes better and more efficient.
Applications of Generative AI in Healthcare
1. Medical Imaging and Diagnostics
A major use case of Generative AI in healthcare is the use of technology in medical imaging. Diagnostic imaging is used to diagnose diseases such as cancer, cardiovascular diseases, and neurological diseases. However, interpreting the images is complex, and it takes time to perform the same. It can be enriched by GenAI, generating high-quality realistic images that help make a more accurate diagnosis. For instance, GenAI solutions in the healthcare sector can combine images from MRI or CT scans to create a single image that provides more information that the physician’s eye can pick and make faster and better decisions.
Another important use of Generative AI in the healthcare sector is to enhance existing images. It can amplify details, filter out extraneous information, or fabricate an image from partial scans, providing the radiologist with a better view of the patient’s situation. This technology will enhance AI’s diagnostic performance in healthcare systems and thus enhance the timely delivery of relevant treatments.
2. Facilitating Drug Discovery and Development
Drug discovery is another field where Generative AI in the healthcare industry is proving to be most helpful. As a process, drug discovery has always taken a long time, often in the range of years, and has been riddled by trial and error. GenAI complements this process by outlining how individual molecules will likely perform, consequently shortening the time it takes to establish optimally performing drug candidates.
Through access to chemical databases and biological networks, GenAI can create new molecules that are likely to help treat diseases. This new approach is also faster and more efficient than the conventional methods of drug development, and the hope is to get lifesaving drugs to market much sooner.
3. Personalized Medicines
Generative AI is applicable in healthcare, but it is most helpful in precision medicine, which delivers treatments unique to every patient’s DNA, lifestyle, and surroundings. Since AI can process large amounts of information concerning a patient’s history, including genetics, it can devise treatment plans that stand a higher chance of working.
For example, artificial intelligence solutions in the healthcare domain can predict a patient’s reaction to a particular treatment based on past clinical history or genetic background. This enables healthcare providers to provide efficient patient care, hence realizing better patient care with minimal adverse effects.
4. Clinical Decision Support
Clinical decision support systems, or CDSS, are tools that assist clinical decision-makers in arriving at better, informed decisions about the kinds of treatment for specific patients. Incorporating Generative AI in healthcare increases the effectiveness of CDSS since it can provide customized advice based on the patient’s health information, disease, and treatment records.
These AI models can forecast the course of a disease, recommend the best course of treatment, and inform doctors and nurses about possible adverse effects or other problems. These aspects make the use of artificial intelligence beneficial in healthcare systems as it yields a high level of decision-making accuracy, which directly impacts the healthcare of the clients.
5. Synthetic Healthcare Data Generation
The application of GenAI in the healthcare industry also solves the problem of data deficiency, which is a widespread problem in many AI healthcare software development solutions. One of the most valuable applications of Generative AI is producing data that looks like patient data but is not linked to a patient. It has the potential for training various AI models and creating new diagnostic tools without violating patient anonymity.
Synthetic data can be used by healthcare providers and researchers to effectively alter some clinical scenarios, train circulating AI systems, or indeed build new datasets when they need them. This approach assists in addressing some privacy issues and makes it possible to build better deep-learning models.
6. Virtual Health Assistants and Chatbots
AI that generates content has led to the creation of smart virtual health assistants and interactive chatbots that can help patients at any time, day or night. These artificial intelligence systems apply natural language processing (NLP) to seek to answer patients’ questions and get back to them with accurate information.
For instance, a chatbot can assist a patient on preoperative instructions, medication regimens or even provide support in the form of words. These virtual assistants lessen the burden on healthcare workers as they take on repetitive tasks, and they enhance the delivery of healthcare.
Benefits of Generative AI in the Healthcare Sector
The adoption of GenAI brings several advantages to the healthcare industry:
-
Improved Efficiency
Automation of activities such as medical image analysis and drug discovery becomes possible due to GenAI to reduce the time and costs required for healthcare delivery. Now, healthcare employees can focus on actual care for patients as AI does the tedious, time-consuming job.
-
Enhanced Accuracy
The ability of AI models to work with extensive data sets to perform complex pattern recognition and increase the rate of predictive analytics. “for example, GenAI may improve the efficiency of imaging in medicine – the so-called diagnostic accuracy – through better image generation. This contributes to better patient health and a lower incidence of medical errors.”
-
Cost Savings
There is a correlation between the reduction of healthcare costs and the speeding up of certain aspects, such as drug development or medical research. Generative AI addresses this challenge by reducing the time required to identify potentially effective drugs and creating synthetic training data, which in turn reduces expenses.
-
Personalized Care
GenAI is able to assist medical staff in formulating treatment strategies based on patient information. Having recourse to AI, care was more personalized to the client’s genetics and past medical records and this means treatment outcomes are much improved, and the client’s satisfaction level is much higher.
Overcoming Challenges in the Adoption of Generative AI
Despite the numerous benefits, the adoption of Generative AI in healthcare comes with its challenges:
1. Data Privacy and Security
Recommendations around clients’ information need to be treated with some caution as the healthcare industry has full control of customers’ private information. While GenAI systems generate images and patterns for patients, typical legal frameworks like the laws associated with HIPAA in the USA restrict the use of specific patient data.
2. Bias in AI Models
The effectiveness of AI systems is determined by the data that has been input into them. AI models can be inaccurate whenever the data is biased, the sample size is small, and when the population is homogeneous. The proper approach is to ensure that such systems are trained on diverse, non-biased datasets if the goal is to eliminate any form of AI bias.
3. Regulatory Concerns
Within the healthcare industry, it is already very clear to stakeholders that applying Generative AI into healthcare does not entail breaching the existing law and regulatory requirements. The formulaic templates are still being produced by governments and regulatory agencies that will control the engagement of AI-based interventions in health conglomerate devices that are deemed to be appropriate for use.
4. Trust and Transparency
Before even considering AI making decisions on behalf of healthcare practitioners, AI should explain the reason behind its actions. This is paramount as people must understand why these models make decisions to be able to trust AI’s role in enhancing patients’ care.
Conclusion
Generative AI possesses numerous capabilities that can be beneficial to the healthcare industry, and this is the reason it has great potential. It has multiple uses in the medical field at present concerning the care of the patient and conducting medical research. There is more and more talk on the use of GenAI in imaging, customizing therapies for patients, and even expediting drug development. GenAI use cases will introduce new technologies or ways of working that will significantly impact the industry. AI will be of great importance in the future of health care because it will minimize the chances of misdiagnosis, assist in providing effective treatments, and even widen the scope of practicing medicine.
So as Generative healthcare AI develops the need for healthcare providers such as clinicians, researchers, and policy makers to focus on potential issues including but not limited to data confidentiality, bias in models, and regulation also increases. Addressing the concerns will assist the integration of Generative AI in healthcare, shaping the future of practicing medicine and improving therapeutic results.
Related Blogs:
Smart Healthcare Through The Internet Of Things Benefits And Applications
HIPAA Compliant Hosting For A Web Application Questions To Ask
Generative AI in Telehealth: Enhancing Telemedicine and Patient Care